What Are the Key Metrics for Evaluating AI Development Services?
In today’s fast-evolving technological landscape, artificial intelligence (AI) has emerged as a game-changer across industries. Businesses increasingly rely on AI development services to gain a competitive edge, optimize processes, and deliver innovative solutions. However, choosing the right AI development partner requires a comprehensive evaluation process. Key metrics can help businesses assess the quality, efficiency, and reliability of these services. This blog explores the critical metrics for evaluating AI development services to ensure they align with your business goals.
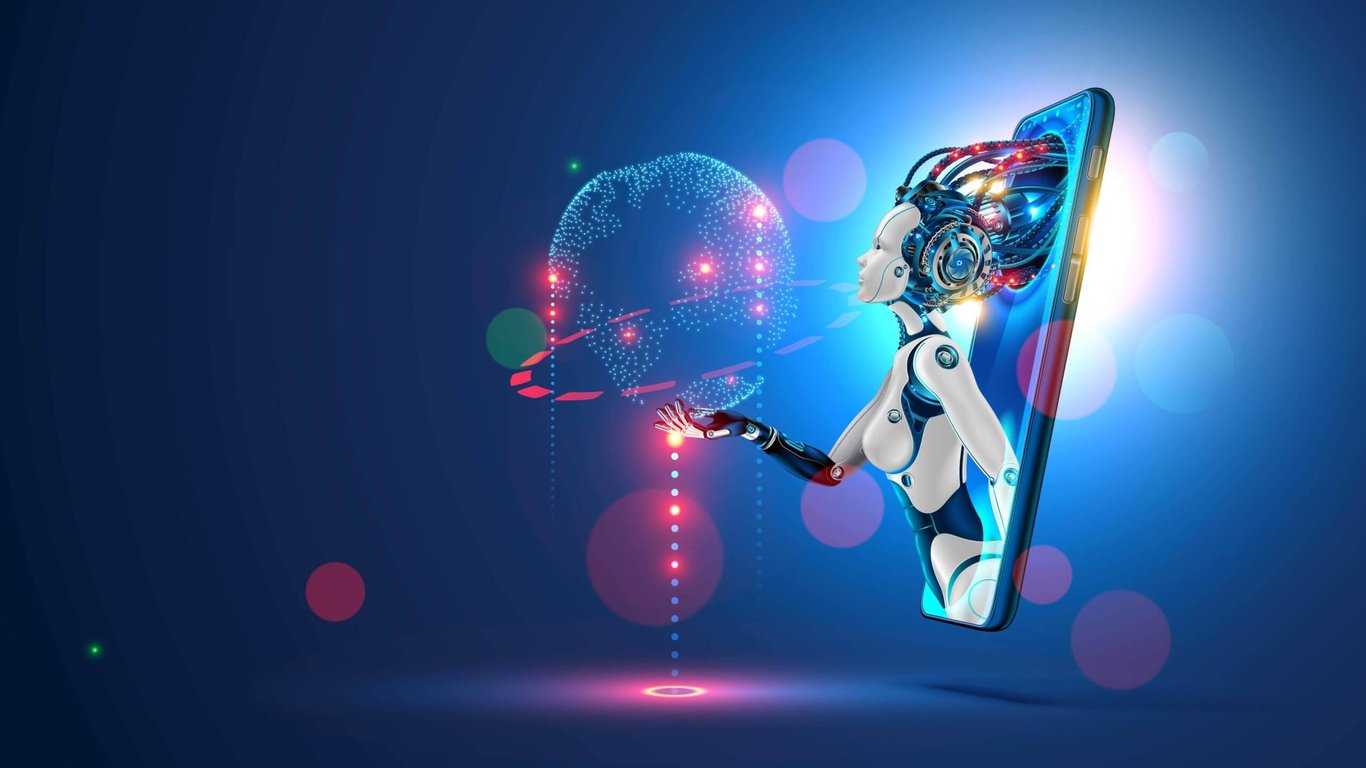
1. Model Accuracy
Accuracy is one of the most fundamental metrics for evaluating AI solutions. It measures how effectively an AI model performs its intended task. For example, in classification tasks, accuracy is the ratio of correctly predicted outcomes to the total predictions made by the model. However, relying solely on accuracy may not provide a complete picture, especially in scenarios where datasets are imbalanced.
Complementary Metrics:
Precision and Recall: These are particularly important for tasks like fraud detection or medical diagnosis, where false positives or false negatives can have significant consequences.
F1 Score: A balanced metric that combines precision and recall to provide a holistic view of model performance.
2. Scalability
Effective AI development Service ensures that an AI solution can scale seamlessly to handle increasing data volumes, rising user demand, and expanded functionality, maintaining optimal performance and adaptability. Scalability ensures that the AI system continues to perform optimally as it handles larger workloads.
Key Questions to Ask:
Can the AI system process larger datasets without compromising performance?
How does the solution integrate with cloud computing or distributed systems for scalability?
3. Processing Speed and Latency
The speed at which an AI system processes data and delivers results is crucial, particularly in real-time applications like fraud detection, recommendation engines, or autonomous systems.
Metrics to Evaluate:
Inference Time: The time taken for the model to make predictions after receiving input.
Throughput: The number of data points processed per second by the model.
4. Cost Efficiency
Cost efficiency involves evaluating the financial investment required for the development, deployment, and maintenance of AI systems. While AI development can be resource-intensive, understanding the cost-benefit ratio helps in determining the value it delivers.
Considerations:
Total Cost of Ownership (TCO): Includes development, infrastructure, licensing, and maintenance costs.
Return on Investment (ROI): Measures the financial benefits derived from the AI solution compared to its costs.
5. Robustness and Reliability
AI systems must be robust enough to handle unexpected inputs or scenarios without failure. Reliability ensures that the AI performs consistently under various conditions.
Evaluation Criteria:
How does the system handle noisy or incomplete data?
Is the system resilient to adversarial attacks?
How often does the system require re-training to maintain performance?
6. Interpretability and Explainability
As AI systems become more complex, understanding their decision-making processes is critical. Interpretability and explainability are essential for building trust, especially in industries like healthcare, finance, and legal sectors.
Metrics to Consider:
Availability of interpretable models or frameworks like SHAP (SHapley Additive exPlanations) and LIME (Local Interpretable Model-Agnostic Explanations).
Ability to generate human-readable explanations for predictions.
7. Integration and Compatibility
AI solutions need to integrate seamlessly with existing systems, workflows, and tools. Compatibility reduces friction during deployment and ensures that the AI system adds value without disrupting operations.
Questions to Evaluate:
Does the AI solution support APIs for easy integration?
How compatible is the system with existing data pipelines, software, and hardware?
8. Data Requirements and Utilization
AI systems rely heavily on data for training and operation. Evaluating how efficiently an AI system utilizes data can indicate its effectiveness.
Metrics to Assess:
Data Efficiency: The amount of data required to achieve desired performance levels.
Data Quality Requirements: The system’s tolerance for noisy, incomplete, or unstructured data.
9. User Experience (UX)
The usability of an AI system is critical, especially for end-user applications. A user-friendly interface and clear outputs enhance adoption and productivity.
Key Aspects:
Is the system intuitive and easy to use?
Does the system provide actionable insights or recommendations?
How much training is required for users to operate the AI system?
10. Ethical and Regulatory Compliance
AI solutions must align with ethical guidelines and regulatory standards to avoid legal and reputational risks. This is especially crucial in industries like finance, healthcare, and public services.
Evaluation Metrics:
Compliance with data privacy laws (e.g., GDPR, HIPAA).
Ethical considerations such as bias mitigation and fairness in decision-making.
Transparency in data usage and AI model training.
11. Maintenance and Support
AI systems require ongoing maintenance to ensure they remain functional and relevant. Assessing the quality of post-deployment support is critical for long-term success.
Questions to Consider:
Does the service provider offer regular updates and improvements?
What is the response time for addressing issues?
Are training and documentation available for in-house teams?
12. Innovation and Adaptability
AI technologies evolve rapidly, and a service provider’s ability to innovate and adapt to new trends is a valuable metric.
Key Indicators:
Frequency of adopting emerging technologies like federated learning or edge AI.
Flexibility in modifying or scaling solutions to meet future requirements.
Conclusion
Evaluating AI development services requires a multidimensional approach, considering technical, operational, financial, and ethical aspects. By focusing on metrics like accuracy, scalability, cost efficiency, and ethical compliance, businesses can make informed decisions when selecting an AI development partner. These metrics not only ensure the successful deployment of AI solutions but also help organizations achieve long-term sustainability and competitive advantage.
喜欢我的作品吗?别忘了给予支持与赞赏,让我知道在创作的路上有你陪伴,一起延续这份热忱!
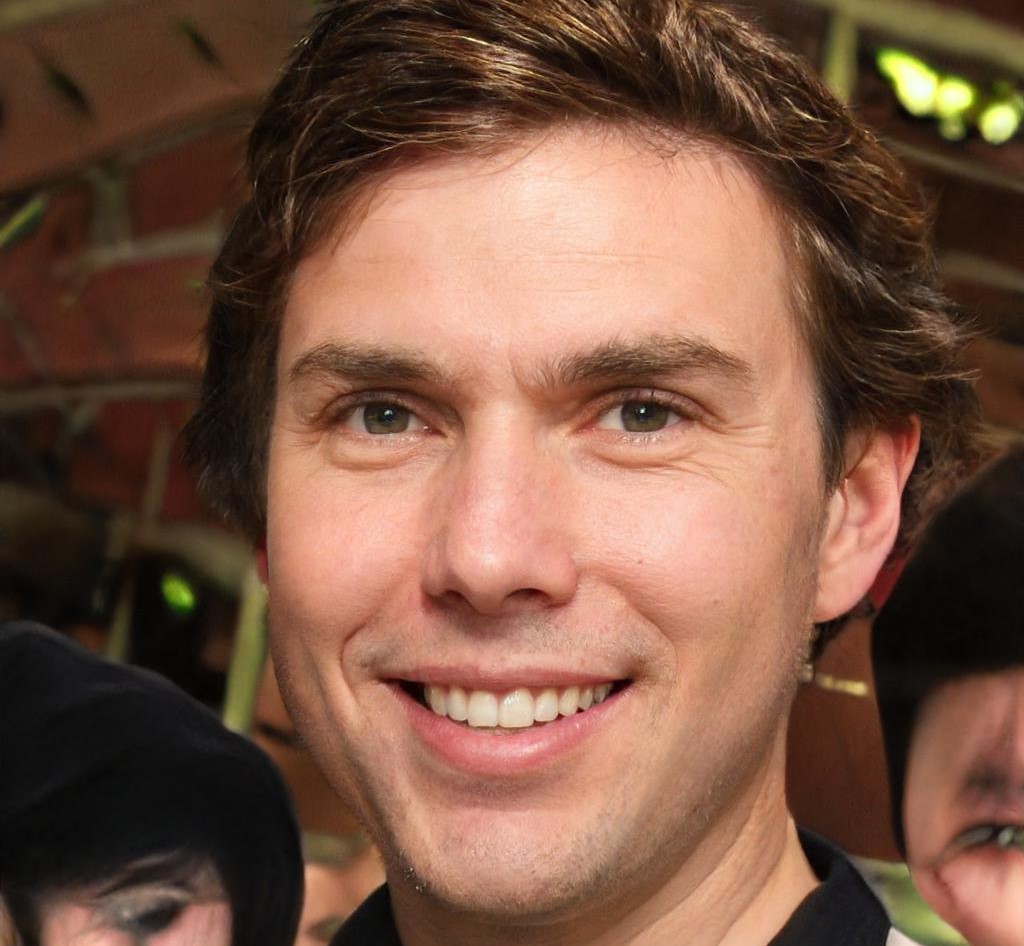
- 来自作者
- 相关推荐